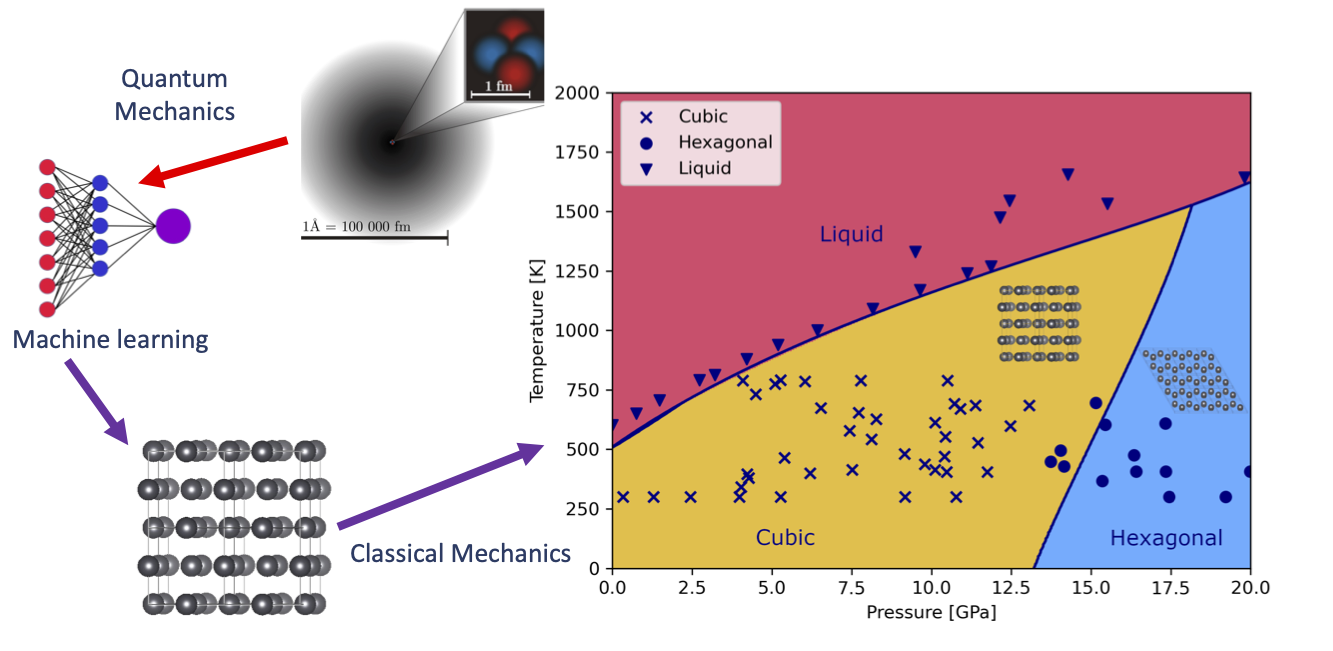
Submitted by Pascal Salzbrenner on Fri, 12/01/2024 - 12:07
Machine learning is quickly gaining prominence in the field of computational materials science. In the Materials Theory Group, we develop so-called ‘machine learned interatomic potentials’ (MLIPs), which can describe the interactions between atoms in both solids and liquids. MLIPs are trained on vast quantities of data generated using accurate quantum mechanical methods. The resulting potentials maintain the accuracy of the underlying model but allow for dramatically faster calculations. A vast array of techniques to perform this task has been developed over the last decade. Ours, the Ephemeral Data-derived Potentials (EDDPs) focuses on simplicity and ease of use.
The EDDPs were originally developed to accelerate crystal structure prediction, with less emphasis initially placed on accuracy for other tasks. In this research paper, we highlight that EDDPs can predict a much wider range of crystal properties as accurately as methods considered state-of-the-art, often at a smaller computational cost. EDDPs yield accelerations of up to 10 million times compared with quantum mechanical methods. This enables calculations which were previously impossible. Properties successfully predicted in this paper span pressure-temperature phase diagrams of heavy metals, fast hydrogen diffusion, and thermal expansion of metal-organic frameworks.
EDDP version 0.2, used to carry out these simulations, is available on the website's Codes page.
Pascal T. Salzbrenner, Se Hun Joo, Lewis J. Conway, Peter I. C. Cooke, Bonan Zhu, Milosz P. Matraszek, William C. Witt, Chris J. Pickard; Developments and further applications of ephemeral data derived potentials. J. Chem. Phys. 14 October 2023; 159 (14): 144801. https://doi.org/10.1063/5.0158710
Image caption: On the right of this image we see a pressure-temperature phase diagram mapping the crystal structures of lead, including its melting curve. The coloured areas are predictions obtained using the EDDPs, the dotted shapes indicate experimental measurements. The left-hand side of the image illustrates the process used to obtain the phase diagram. Starting from quantum-mechanical data, machine learning is used to create an interatomic potential. With this potential, classical mechanics simulations are run to predict structural stability. This can be done in an afternoon on a modern laptop. If quantum mechanical methods were used directly, the required time would instead be around 300 years.