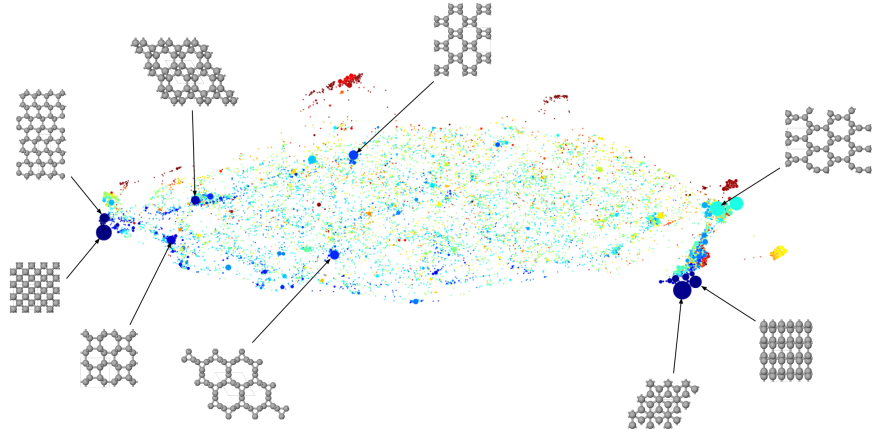
Submitted by Chuck Witt on Thu, 25/11/2021 - 16:20
Computational structure prediction has emerged as a highly successful approach to the discovery of new materials. Candidate structures are created by constructing the most stable configurations that can be adopted by a given set of atomic building blocks. This corresponds to finding the deepest regions of an energy landscape, which is defined by the variation of a system’s energy with the relative positions of its atoms. The development of efficient methods for exploring these landscapes requires a detailed understanding of their topologies. However, these surfaces live in spaces with very large numbers of dimensions, making them difficult to visualize. In this work, Ben Shires and Chris Pickard introduce an approach to visualizing the distribution of stable structures across a high-dimensional energy landscape, based on machine-learning algorithms for dimensionality reduction.
The new approach is applied to well-studied model systems (clusters of Lennard-Jones particles) and to datasets of realistic solid-state structures (configurations of carbon and the multicomponent system C+H+N+O). Results reveal known features of these energy landscapes as well as previously undiscovered ones, demonstrating the insight that the method can provide. A key finding is that the distribution of stable structures through the energy landscape can be low dimensional even though the entire surface is not.
Visualizing Energy Landscapes through Manifold Learning
Benjamin W. B. Shires and Chris J. Pickard
Physical Review X 11, 041026 (2021)